Ananta R. Bhattarai
Ph.D. researcher at Bielefeld University.
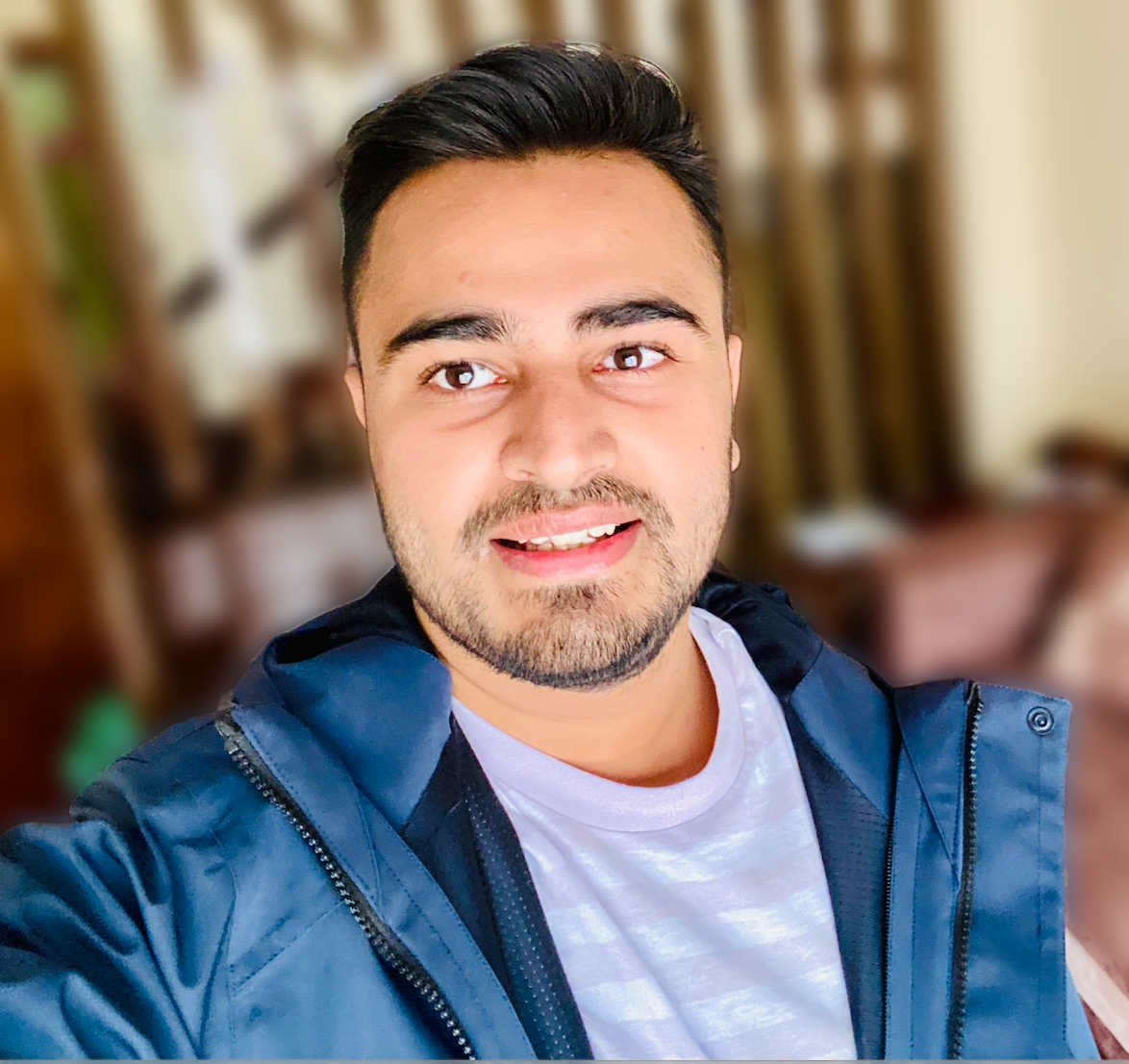
Hi, I am Ananta. I am a Ph.D. student at Bielefeld University (Visual AI for Extended Reality Lab led by Prof. Helge Rhodin). I completed my Master’s degree in Computer Science from TU Munich advised by Prof. Matthias Niessner and Artem Sevastopolsky. Alongside my Master’s studies, I was fortunate to work with Dr. Michael Lebacher and Prof. Florian Buettner on Explainable AI at Siemens AG.
Previously, I completed my Bachelor’s degree in Computer Science at Jacobs University Bremen. During my Bachelor’s, I spent a term abroad at Carnegie Mellon University, where I did independent research under the supervision of Prof. Ruslan Salakhutdinov.
I’m interested in working at the intersection of 3D computer vision, computer graphics, and machine learning.
news
Sep 01, 2024 | Started my Ph.D. at Bielefeld University with Prof. Helge Rhodin. |
---|---|
Jun 30, 2024 | A paper is accepted at ICML 2024. |
Oct 30, 2023 | TriPlaneNet is accepted at WACV 2024. |
Aug 30, 2023 | Successfully defended my Master’s thesis on 3D GAN Inversion with Deep Learning. |
Apr 30, 2023 | A paper is accepted at SAFECOMP 2023. |
selected publications
- SAFECOMP23Towards Scenario-Based Safety Validation for Autonomous Trains with Deep Generative ModelsInternational Conference on Computer Safety, Reliability, and Security (SAFECOMP), 2023
- AAAI21Combining Programmable Potentials and Neural Networks for Materials ProblemsAAAI Spring Symposium: MLPS, 2021